In the ever-expanding landscape of artificial intelligence (AI), one critical aspect that demands immediate attention is building trust.
As AI systems become increasingly integrated into various aspects of our lives, from autonomous vehicles to virtual assistants, trust in their decision-making abilities and ethical conduct becomes paramount. Addressing this concern, the concept of AI alignment emerges as a promising approach to fostering trust in AI.
AI alignment refers to the process of aligning AI systems’ goals and behaviors with human values and intentions. By ensuring that AI systems understand and act in accordance with our ethical principles, we can cultivate trust in their actions and outcomes. Achieving AI alignment is a complex endeavor that requires meticulous attention to detail, rigorous ethical frameworks, and advanced technological solutions.
In these case studies, we delve into the multifaceted realm of fostering trust through AI alignment. We explore the significance of aligning AI systems with human values, examine the challenges and considerations involved, and highlight potential strategies and techniques to facilitate trustworthy AI. Through a comprehensive understanding of AI alignment’s role in building trust, we can pave the way for responsible and reliable AI systems that positively impact society.
Case-Study: Ethical AI and Human Values
I. Use Case Description
Financial institutions recognize the immense potential of AI systems in transforming their risk assessment and decision-making processes. By leveraging the power of AI, these institutions aim to improve the accuracy and efficiency of their operations. However, in the pursuit of automation and optimization, it becomes imperative to align these AI systems with human values, ensuring fairness, non-discrimination, and transparency in their outcomes. This use case places a strong emphasis on addressing these critical concerns by integrating fairness-aware algorithms, establishing continuous monitoring mechanisms for bias, and delivering clear explanations for decision outcomes. The primary goal is to counteract biases that may arise within the credit scoring domain and cultivate trust among stakeholders within financial processes.
Firms have increasingly turned to AI systems to streamline their risk assessment and decision-making procedures. These systems, driven by sophisticated algorithms, have the potential to analyze vast amounts of data, identify patterns, and make predictions with remarkable accuracy. Such capabilities offer the promise of improving credit scoring mechanisms, enabling lenders to make informed decisions regarding loan approvals, interest rates, and credit limits. However, it is essential to recognize that these AI systems are not immune to biases that may exist within historical data or the algorithms themselves.
Fairness-Aware Algorithms
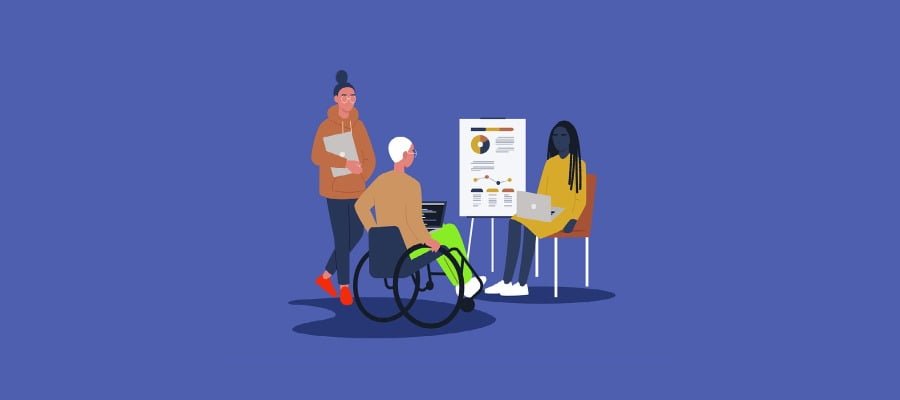
To address these concerns, the use case focuses on incorporating fairness-aware algorithms into the AI systems utilized by financial institutions. These algorithms are designed to proactively identify and mitigate biases, ensuring that the credit scoring process is conducted in a fair and unbiased manner. By accounting for protected attributes such as race, gender, age, or ethnicity, these fairness-aware algorithms strive to eliminate discriminatory practices and provide equal opportunities to all individuals, regardless of their background.
Moreover, the use case emphasizes the need for continuous monitoring of the AI systems to detect any emerging biases or patterns of discrimination. This ongoing monitoring serves as a proactive measure to safeguard against unintended biases that may arise over time due to shifts in societal dynamics or changes in the data being used. By regularly assessing the fairness metrics and performance of the AI models, financial institutions can take corrective actions promptly, ensuring that their systems remain aligned with human values and regulatory requirements.
In addition to bias mitigation, the use case recognizes the importance of transparency and explainability in financial decision-making processes. To build trust and confidence among stakeholders, the AI systems employed by financial institutions are designed to provide clear and comprehensible explanations for the factors influencing the decision outcomes. Customers and other relevant parties can gain insights into how their creditworthiness is assessed, what variables contribute to the decisions made, and how different factors are weighted within the algorithms. This transparency not only promotes understanding but also allows individuals to challenge or seek clarification on decisions, fostering a sense of accountability and fairness.
II. Actors
Financial institution: The organization utilizing AI systems for risk assessment and decision-making in their financial processes. Financial institutions, such as banks, credit unions, and lending organizations, heavily rely on AI systems to analyze vast amounts of data and make informed decisions regarding credit scoring, loan approvals, and other financial assessments. These institutions aim to enhance their operational efficiency, reduce risks, and provide accurate and timely financial services to their customers. They actively seek to leverage AI technologies and ensure the alignment of their systems with human values, including fairness, non-discrimination, and transparency. Financial institutions also play a pivotal role in establishing partnerships with data scientists and collaborating with regulators to comply with industry regulations and standards.
Data scientists: Experts responsible for designing and implementing AI algorithms and models. Data scientists are highly skilled professionals with expertise in machine learning, statistics, and data analysis. They play a crucial role in developing and refining the AI algorithms and models used in financial risk assessment. These experts work closely with financial institutions to understand their specific needs and objectives, translating them into algorithmic solutions. They are responsible for collecting, preprocessing, and cleaning the data, identifying relevant features, and training the AI models. Data scientists also ensure the fairness and non-discrimination of the AI systems by incorporating fairness-aware algorithms and regularly evaluating the models’ performance. They continuously monitor for biases, address ethical concerns, and strive to improve the accuracy and reliability of the AI systems used in financial risk assessment.
Regulators: Authorities monitoring the compliance of financial institutions with fair lending practices and regulations. Regulators, such as government agencies and central banks, are responsible for overseeing and enforcing regulations that govern financial institutions’ operations. They play a critical role in maintaining a fair and transparent financial ecosystem by ensuring that financial institutions comply with legal requirements, industry standards, and ethical guidelines. Regulators monitor the practices of financial institutions, including their use of AI systems for risk assessment and decision-making. They assess whether these institutions adhere to fair lending practices, avoid discrimination, and provide transparent explanations for their decisions. Regulators also work collaboratively with financial institutions and other stakeholders to establish guidelines and frameworks that promote fairness, non-discrimination, and the responsible use of AI in financial processes.
UX Highlight
The best part of our job is helping people create digital journeys.
III. Goals
Mitigate biases in credit scoring: The use of AI systems should minimize biases related to protected attributes such as race, gender, age, or ethnicity in the credit scoring process.
Ensure fairness and non-discrimination: The AI systems should treat all individuals equally and avoid any form of discrimination based on protected attributes.
Enhance transparency and explainability: The decision-making process of AI systems should be transparent and explainable, allowing stakeholders to understand the factors influencing the decisions made.
Foster trust in financial processes: By addressing biases, ensuring fairness, and providing explanations, AI systems aim to build trust between the financial institution and its customers.
IV. Preconditions
Availability of historical data: Sufficient and diverse historical data related to credit scoring is available for training and evaluating the AI models.
Access to relevant features: The necessary features and variables required for risk assessment, including both financial and non-financial factors, are accessible.
Compliance with legal and regulatory frameworks: The financial institution operates within the guidelines and regulations established by relevant authorities.
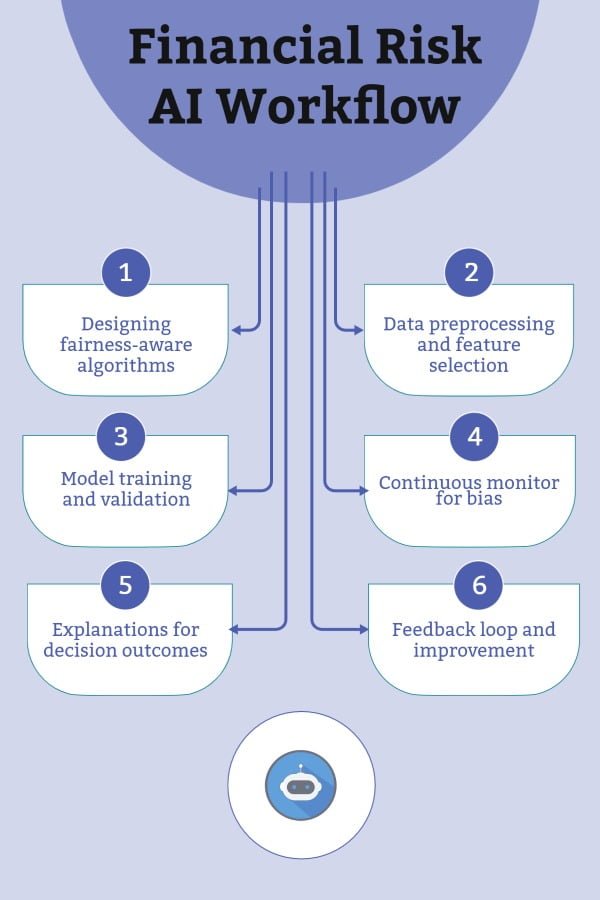
Click to expand description
Data scientists develop and implement AI algorithms that account for fairness, avoiding biases in the credit scoring process. These algorithms consider protected attributes as well as other relevant factors to ensure fair and unbiased risk assessment.
The historical data is preprocessed to identify and address any biases present. Data scientists carefully select features that are relevant to risk assessment while avoiding variables that may introduce discrimination or biases.
Using the preprocessed data, the AI models are trained, evaluated, and validated. Various fairness metrics are utilized to assess and monitor the models’ performance in terms of avoiding discrimination and biases.
The deployed AI systems continuously monitor the decision outcomes to identify any biases or discriminatory patterns. Regular fairness audits are conducted to ensure ongoing alignment with human values and compliance with fair lending regulations
The AI systems are equipped with explainability mechanisms that provide transparent and interpretable insights into the factors influencing the decision outcomes. Customers and stakeholders can understand why a particular decision was made, enhancing trust and reducing uncertainty.
Feedback from customers, regulators, and other stakeholders is collected and analyzed to identify potential areas of improvement. The AI models are iteratively refined to further enhance fairness, transparency, and trust in the financial risk assessment processes.
Alternative Flows
In the event that biases or discriminatory patterns are identified during the continuous monitoring of the AI systems, financial institutions take proactive measures to rectify these issues and promote fairness in their risk assessment and decision-making processes. Upon detecting such biases, the institutions initiate a process of retraining the AI models using updated data and algorithmic improvements. This entails analyzing the root causes of the biases, understanding the factors that contribute to them, and devising strategies to mitigate their impact.
To address biases, financial institutions collaborate with data scientists and subject matter experts to evaluate the existing AI models and identify areas where improvements can be made. They may conduct an in-depth analysis of the training data, examining the representation of different demographic groups and protected attributes to ensure that all individuals are treated fairly. By augmenting the training data with additional samples from underrepresented groups or adjusting the weighting of certain features, financial institutions strive to eliminate biases and achieve a more accurate and unbiased risk assessment process.
In parallel, algorithmic improvements are made to enhance the fairness of the AI models. Data scientists work on refining the algorithms, modifying the decision-making logic, or introducing additional fairness constraints to reduce the potential for biases in the system. These improvements are based on rigorous evaluation and validation processes, ensuring that the updated models demonstrate improved performance in terms of fairness, accuracy, and non-discrimination.
Additionally, financial institutions are cognizant of the evolving regulatory landscape and the potential introduction of new requirements or guidelines. They actively monitor changes in regulations related to fair lending practices and AI usage, ensuring compliance and alignment with the new standards. If additional regulatory requirements or guidelines are introduced, the AI systems and processes are adjusted accordingly to meet the updated compliance obligations. This may involve revisiting the AI models, incorporating new regulatory guidelines into the algorithmic design, or adapting the data collection and handling practices to meet the evolving regulatory expectations.
Financial institutions understand the importance of adapting their AI systems to reflect changes in societal expectations, ethical considerations, and regulatory frameworks. They invest resources in staying informed about emerging best practices, industry standards, and legal requirements to maintain the integrity and fairness of their financial risk assessment processes. By proactively addressing biases, continuously improving the algorithms, and staying compliant with regulations, financial institutions ensure that their AI systems are aligned with the highest standards of fairness, transparency, and non-discrimination.
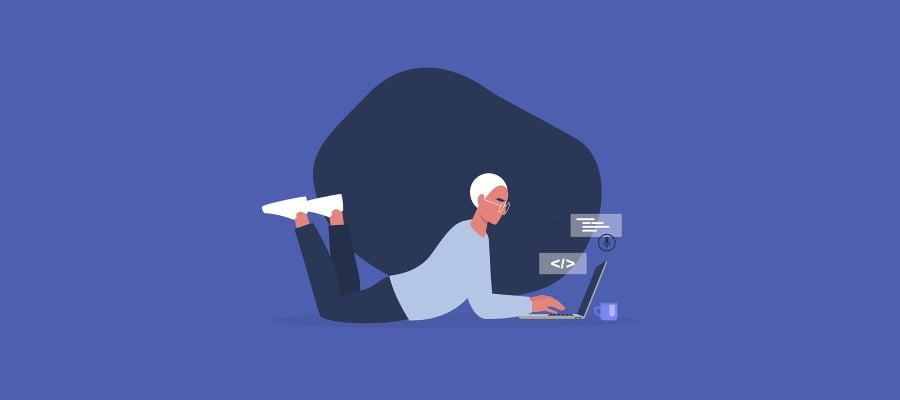
Postconditions
- The financial institution utilizes AI systems that mitigate biases, ensure fairness, and enhance transparency in its risk assessment and decision-making processes.
- Stakeholders, including customers and regulators, have increased trust and confidence in the financial institution’s practices, fostering stronger relationships.
Exceptions
Lack of diverse and representative data: If the historical data used for training the AI models is not diverse or representative, it may introduce biases or fail to capture the full range of relevant factors. In such cases, efforts should be made to augment the data or consider alternative data sources to ensure fairness and accuracy.
Ethical considerations: In situations where the AI systems are designed to optimize financial outcomes without considering ethical implications, there is a risk of disregarding human values and societal impact. It is essential to incorporate ethical guidelines and considerations into the design and decision-making processes to avoid negative consequences.
Limitations of explainability: While efforts are made to provide explanations for decision outcomes, there may be instances where the complexity of the AI models makes it challenging to provide clear and comprehensive explanations. In such cases, transparency should be maintained by highlighting the limitations and providing as much insight as possible.
Stakeholders
- Financial institution management: Responsible for overseeing the implementation and monitoring of the AI systems and ensuring alignment with the institution’s values and strategic goals.
- Data scientists and AI experts: Involved in developing, training, and fine-tuning the AI models, as well as continuously monitoring for biases and improving the system’s performance.
- Customers and applicants: Individuals seeking financial services who are impacted by the decisions made by the AI systems. Their trust and satisfaction are crucial for the success of the risk assessment process.
- Regulators and compliance officers: Authorities responsible for ensuring fair lending practices and compliance with relevant regulations. They play a role in reviewing the AI systems and monitoring the financial institution’s adherence to fair lending standards.
- Internal auditors and risk management teams: Responsible for conducting audits, risk assessments, and monitoring the performance and effectiveness of the AI systems in reducing biases and ensuring fairness.

Related Use-Cases
Customer Support Enhancement: AI systems can be utilized to improve customer support processes, providing personalized and efficient assistance in areas such as loan applications and credit inquiries, while ensuring fairness and non-discrimination.
Fraud Detection and Prevention: AI systems can be employed to detect and prevent fraudulent activities in financial transactions, using advanced algorithms to identify suspicious patterns and behaviors while maintaining fairness and transparency.
Compliance Monitoring and Reporting: AI systems can assist in monitoring and reporting compliance with regulatory requirements, ensuring that financial institutions adhere to fair lending practices and providing transparency in their decision-making processes.
Risk and Mitigation
Data biases: Biases present in historical data used for training AI models have the potential to perpetuate discriminatory practices and reinforce existing inequalities. To mitigate this risk, financial institutions employ a multi-faceted approach. It begins with careful preprocessing of the data, where thorough analysis and cleansing techniques are applied to identify and address any biases present. Additionally, data augmentation techniques may be employed to enhance the representativeness and diversity of the training data. This involves synthesizing or augmenting data points to ensure fair and comprehensive coverage of different demographic groups and protected attributes. Furthermore, ongoing monitoring for biases is crucial to identify any emerging patterns or unintended discriminatory effects. Regular assessments and audits of the data quality and biases within the AI models are conducted, enabling institutions to take corrective measures promptly and ensure fair and unbiased risk assessment outcomes.
Over-reliance on AI: While AI systems bring numerous benefits to financial risk assessment, there is a risk of over-reliance on these systems without adequate human oversight. This can lead to decision-making processes that disregard contextual factors and ethical considerations. To mitigate this risk, financial institutions implement regular human audits and reviews of the AI systems and the decisions they produce. Human experts with domain knowledge and ethical expertise conduct independent assessments of the system’s outputs, ensuring that the decisions align with human values, legal requirements, and ethical guidelines. These audits provide an essential layer of accountability, enabling human judgment and consideration of nuanced factors that may not be captured by AI algorithms alone. By incorporating human oversight, financial institutions strike a balance between the efficiency and accuracy of AI systems and the necessity of human judgment in complex decision-making processes.
Lack of transparency: Inadequate transparency in explaining the factors influencing decisions made by AI systems can erode trust among stakeholders. To mitigate this risk, financial institutions prioritize the incorporation of explainability mechanisms into their AI systems. These mechanisms aim to provide clear and understandable explanations for decision outcomes. Techniques such as interpretable machine learning models, rule-based systems, or algorithmic feature importance analysis are employed to shed light on the factors that contribute to the decisions made by AI systems. Transparent explanations help customers, regulators, and other stakeholders to understand the rationale behind the decisions, instilling confidence and trust in the financial processes. Additionally, financial institutions communicate and disclose their approach to decision-making, including the criteria, variables, and considerations taken into account, further enhancing transparency and accountability.
Adversarial attacks: AI systems are vulnerable to adversarial attacks that aim to manipulate decision outcomes for malicious purposes. Financial institutions recognize this risk and employ robust security measures to mitigate potential attacks. These measures involve incorporating advanced cybersecurity protocols, encryption techniques, and access controls to safeguard the AI systems and the data they process. Additionally, continuous model monitoring is essential to detect and mitigate adversarial attacks. Regular assessments of the model’s performance, robustness, and vulnerability to potential threats are conducted. This includes stress-testing the AI systems against various attack scenarios to identify vulnerabilities and implement necessary safeguards. By adopting a proactive security stance and maintaining continuous monitoring, financial institutions minimize the risk of adversarial attacks and ensure the integrity and reliability of their AI systems in financial risk assessment.
Free UI Design Courses
Conclusion
The integration of fairness-aware algorithms, continuous monitoring for bias, and transparent explanations for decision outcomes in AI systems for financial risk assessment hold significant potential to align these systems with human values and promote ethical practices within the financial sector. By prioritizing fairness and non-discrimination, financial institutions can foster trust, enhance customer relationships, and establish a more equitable financial landscape. The incorporation of fairness-aware algorithms is a crucial step toward eliminating biases and promoting equal treatment of individuals in the risk assessment process. These algorithms are designed to recognize and mitigate biases associated with protected attributes such as race, gender, age, or ethnicity, ensuring that these factors do not influence decisions unfairly. By incorporating considerations of fairness into the algorithms, financial institutions are taking proactive measures to promote equality of opportunity and reduce systemic discrimination.
In addition, continuous monitoring for bias is vital to ensure the ongoing fairness and non-discrimination of AI systems. Financial institutions employ mechanisms to regularly assess the performance of their AI models and detect any emerging biases or patterns of discrimination. This monitoring allows for timely interventions and adjustments, enabling institutions to address biases promptly and maintain alignment with human values. By actively monitoring for bias, financial institutions demonstrate their commitment to fair and equitable practices, building trust among customers and stakeholders. Transparent explanations for decision outcomes play a crucial role in building trust and promoting understanding in financial risk assessment processes. Customers and stakeholders have the right to know why certain decisions were made and how factors, both financial and non-financial, influenced those decisions. Financial institutions equip their AI systems with explainability mechanisms that provide clear and interpretable insights into the factors considered in the decision-making process. This transparency helps customers understand the basis of their financial assessments, fostering trust and confidence in the fairness and integrity of the system.
The alignment of AI systems with human values in financial risk assessment yields numerous benefits. Trust is a fundamental element in the customer-institution relationship, and when financial institutions prioritize fairness and non-discrimination, they build stronger and more positive relationships with their customers. Customers feel valued and respected, knowing that their financial assessments are conducted in a fair and unbiased manner. By aligning with human values, financial institutions enhance their reputation, attract a broader customer base, and contribute to a more inclusive and equitable financial ecosystem. Ultimately, the incorporation of fairness-aware algorithms, continuous monitoring for bias, and transparent explanations for decision outcomes in AI systems represents a significant step toward ethical and responsible financial risk assessment. Financial institutions that prioritize these values demonstrate their commitment to fairness, equality, and non-discrimination, fostering trust, building stronger relationships, and contributing to a more equitable financial landscape for all.